Agriculture is one of the oldest and most essential industries, responsible for sustaining the world’s population. However, as the global population grows and resources become increasingly scarce, the agricultural sector faces significant challenges in meeting the rising demand for food. This predicament has prompted the integration of advanced technologies, such as machine learning, into agricultural practices, revolutionizing the way we approach crop productivity and efficiency.
Machine learning, a subset of artificial intelligence, is a powerful tool that enables computers to learn from data, identify patterns, and make predictions without being explicitly programmed. By harnessing the capabilities of machine learning algorithms, farmers and agricultural experts can unlock new avenues for optimizing crop yields, minimizing resource wastage, and improving overall farm management.
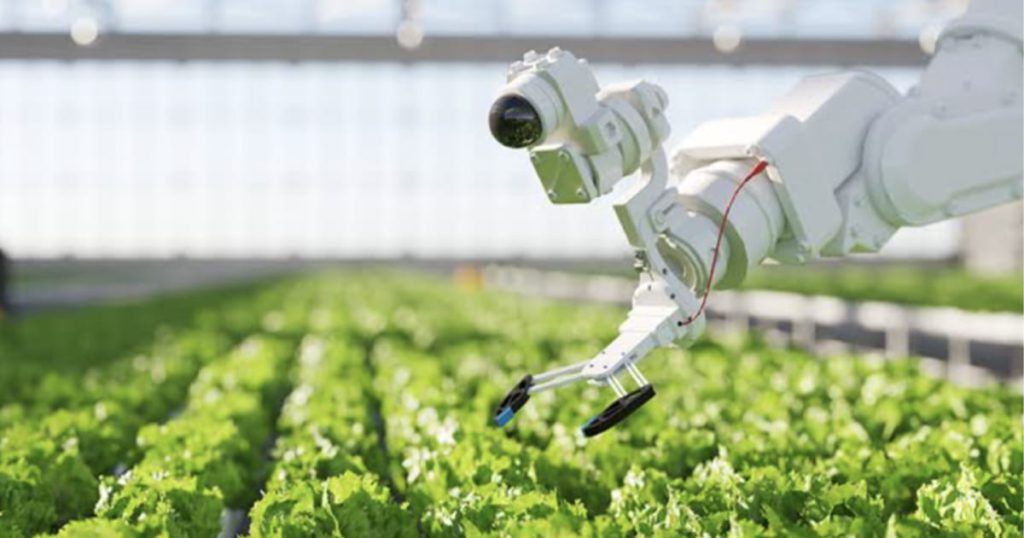
Precision Agriculture and Yield Optimization
One of the most significant applications of machine learning in agriculture is precision farming. This approach involves collecting and analyzing vast amounts of data from various sources, including satellite imagery, soil sensors, weather stations, and historical yield records. Machine learning algorithms can process this data and provide valuable insights into soil fertility, crop health, moisture levels, and other critical factors that influence crop growth.
By leveraging these insights, farmers can make informed decisions about crop selection, planting times, irrigation schedules, and fertilizer applications, tailored to the specific needs of their fields. This level of precision not only maximizes crop yields but also reduces input costs, such as water and fertilizers, leading to increased profitability and sustainability.
Predictive Analytics and Risk Management
Agriculture is inherently susceptible to various risks, including adverse weather conditions, pest infestations, and plant diseases. Machine learning algorithms can analyze historical data, along with real-time environmental factors, to predict potential threats and provide early warning systems. This proactive approach enables farmers to take preventive measures, such as adjusting irrigation schedules, applying targeted pesticides, or implementing crop rotation strategies, thereby minimizing crop losses and mitigating risks.
Furthermore, predictive analytics can assist in forecasting market trends, demand patterns, and pricing fluctuations. This empowers farmers to make informed decisions regarding crop selection, storage, and marketing strategies. By anticipating market dynamics, farmers can optimize their operations and maximize their profitability.
Also Read:- What is Green Manure, Its Types, and Benefits?
Automated Monitoring and Decision Support Systems
Traditional agricultural practices often rely on manual labor and human observation, which can be time-consuming, error-prone, and limited in scale. Machine learning algorithms can automate various monitoring and decision-making processes, reducing the burden on human resources and enabling more efficient farm management.
For instance, computer vision techniques can be employed to analyze images and videos captured by drones or field cameras, enabling real-time monitoring of crop health, detecting pest infestations, and identifying areas requiring immediate attention. Machine learning models can then recommend appropriate actions, such as targeted pesticide application or localized irrigation adjustments, minimizing waste and maximizing resource efficiency.
Moreover, decision support systems powered by machine learning can assist farmers in making informed choices regarding crop rotations, fertilizer applications, and harvest times, taking into account a multitude of factors, including historical data, weather patterns, and market trends.
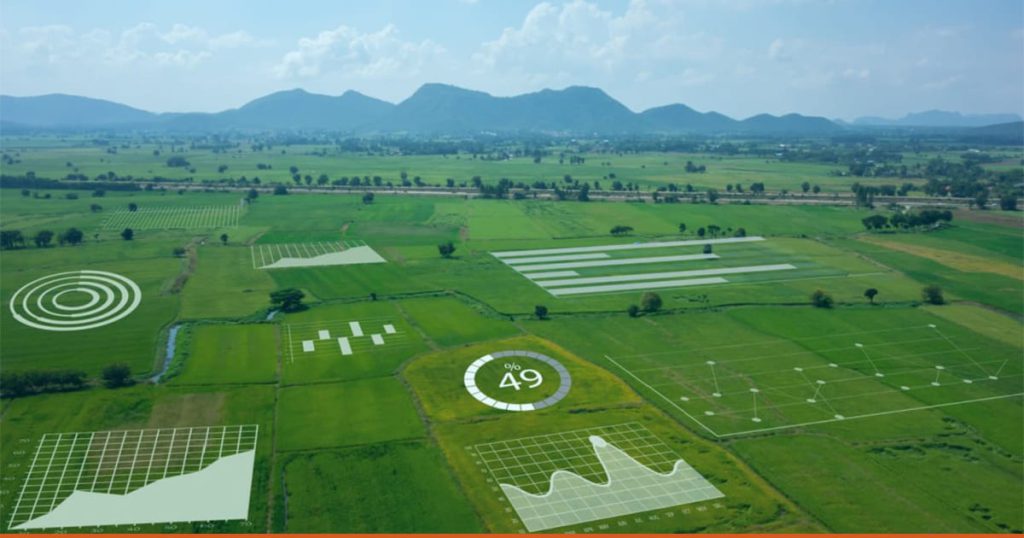
Robotics and Automation
The integration of machine learning with robotics and automation technologies is transforming agricultural practices, particularly in the areas of planting, harvesting, and crop maintenance. Autonomous robots equipped with machine learning capabilities can navigate fields, identify individual plants, and perform tasks such as precision seeding, targeted spraying of pesticides or fertilizers, and selective harvesting.
These robotic systems can operate with greater accuracy, consistency, and efficiency compared to traditional manual methods, reducing labor costs and minimizing waste. Additionally, machine learning algorithms can optimize the paths and movements of these robots, further enhancing their productivity and resource utilization.
Breeding and Genetic Optimization
Machine learning techniques are also being applied to plant breeding and genetic optimization. By analyzing vast datasets of genetic information, phenotypic traits, and environmental factors, machine-learning algorithms can identify desirable genetic markers and assist in developing crop varieties that are more resistant to pests, diseases, and environmental stresses.
This approach not only improves crop yields but also contributes to long-term sustainability by reducing the reliance on pesticides and increasing resilience to climate change. Furthermore, machine learning can accelerate the breeding process by identifying promising genetic combinations more efficiently, reducing the time and resources required for traditional breeding methods.
Despite the numerous benefits offered by machine learning in agriculture, its widespread adoption faces several challenges. These include the availability and quality of data, the complexity of integrating diverse data sources, and the need for substantial computational resources and expertise. Additionally, concerns surrounding data privacy, algorithmic bias, and the potential displacement of human labor must be addressed to ensure responsible and ethical implementation.
Conclusion
Nevertheless, the potential of machine learning to revolutionize agriculture is undeniable. By leveraging the power of data and advanced algorithms, farmers can make informed decisions, optimize resource utilization, and enhance crop productivity and efficiency. As the world’s population continues to grow and environmental challenges intensify, the integration of machine learning into agricultural practices will play a crucial role in ensuring food security, sustainability, and resilience in the face of global challenges.